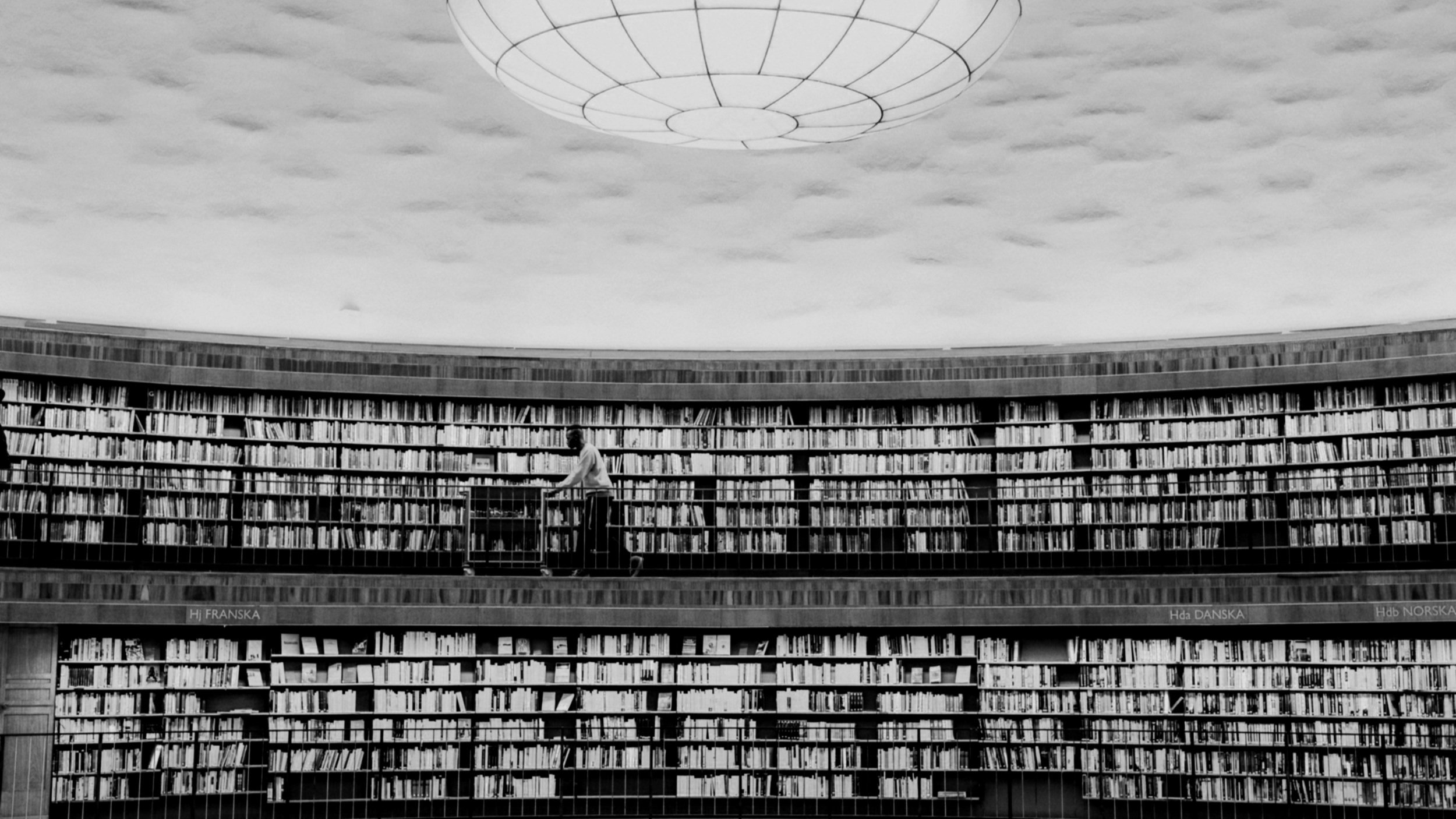
LEarn
This is a resource repository for legislators, policy makers, journalists, thought leaders, and researchers. Artificial intelligence can be confusing and overwhelming. We aim to provide clarity and understanding. The modules, articles, and guides presented here are intended to explain fundamental concepts in artificial intelligence and AI governance in accurate and non-technical language. New articles will be added as the technology and language of AI evolve—and they’re evolving quickly.
To learn more about the Transparency Coalition’s top remedies for current risks in AI safety and transparency, see our Solutions page.
topics
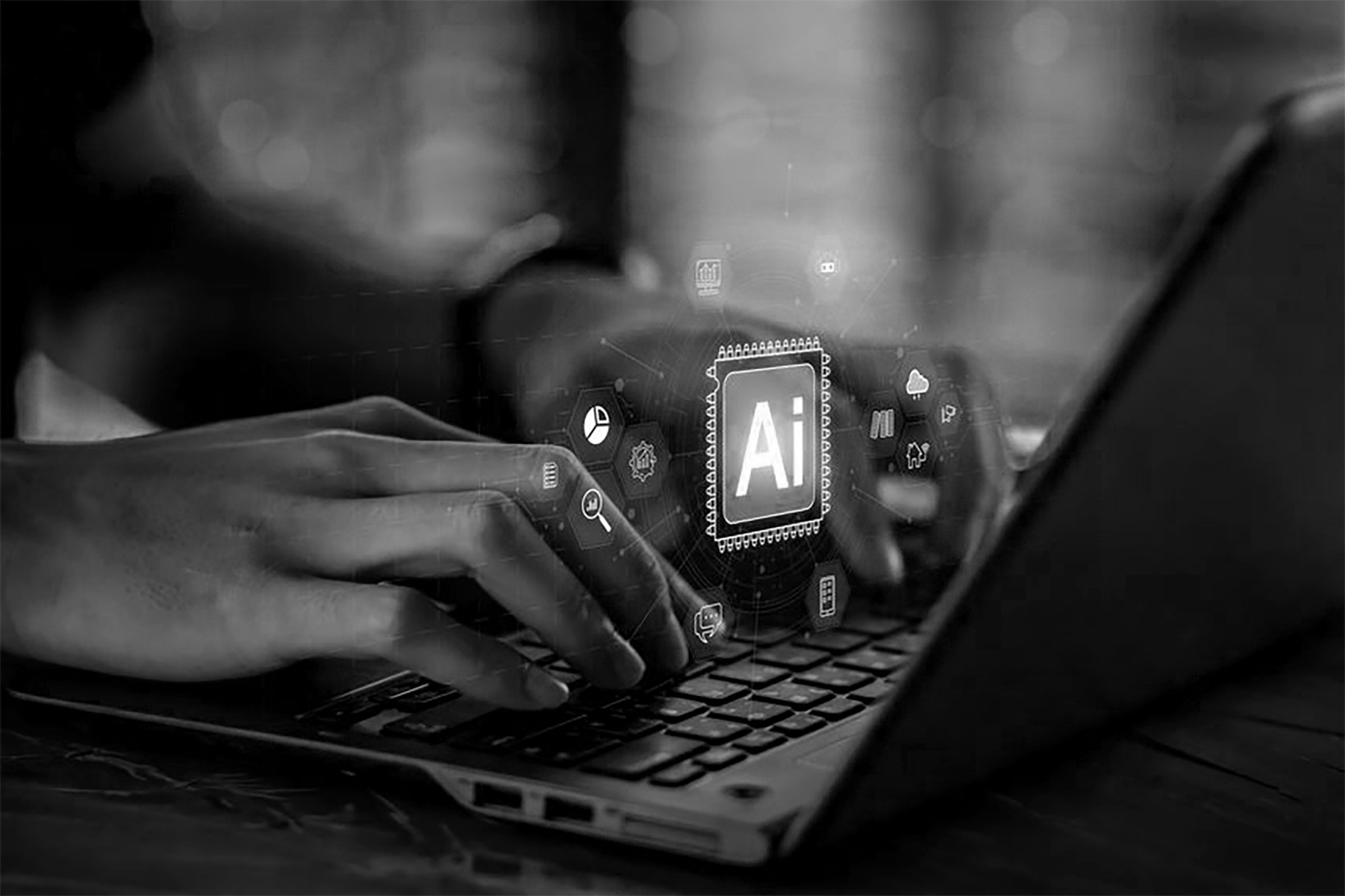
Read our Latest AI Report
TCAI Advisor Leigh Wickell unearths the roots of today’s outdated privacy laws, and sets a course for an AI-era update
AI 101
Your startup guide on the most essential concepts for understanding Artificial Intelligence.
AI Safeguards
Exploring the foundations of AI safeguards and mitigation.
Training Data transparency
Learn about the foundational ingredients of AI models, and why and how they should be disclosed.
DISCLOSING AI USE
Understand the importance of AI disclosure laws, and how content provenance makes disclosure possible.
Synthetic Data 101
Learn about the difference between organic data and synthetic data, and how it affects AI performance.
Data Privacy in AI
How and why AI presents new challenges in data privacy.
TCAI report
Privacy Harms in the AI Age takes an in-depth look at America’s outdated privacy laws and offers solutions for the emerging AI landscape.
Select image at left to download the full report.
TCAI research: further resources
TCAI guides to AI lawsuits, state data privacy laws, and more.
Complete Resource Library
TCAI Guide to Search Tools: Was Your Data Used to Train an AI Model?
Search engines have emerged recently that allow individuals to check specific types of content—books and images—for use as AI training data.
We link to the search tools, and include tips on preventing your data from being used to train AI models.
AI Safeguards: Where to Start
At the Transparency Coalition we believe AI policy discussion and legislative action happen at many levels simultaneously. Our mission is to address known AI safety and privacy risks with practical solutions. We’re focused on bringing transparency to both AI inputs and AI outputs.
Input Safeguards: Require Transparency in AI Training Data
Transparency in AI training data is the foundation of ethical AI.
State legislatures should consider measures that require developers of AI systems and services to publicly disclose specified information related to the datasets used to train their products.
TCAI Guide to AI Lawsuits
The hailstorm of AI-related lawsuits over the past year can make the litigation space feel chaotic and confusing. In fact, the lawsuits can be roughly sorted into two buckets: copyright infringement and harmful AI-driven outcomes.
This TCAI curated guide offers a clear and concise overview of today’s AI legal battlefield.
TCAI Guide to State Data Privacy Laws
The United States has no national data privacy law.
In the absence of a national regulatory mechanism, many individual states have adopted their own digital privacy laws to protect their citizens from the misuse of personal data.
We’ve gathered information on individual state laws, as well as national and local bills, in this TCAI guide.
Understanding Synthetic Data
In today’s AI ecosystem there are two general types of training data: organic and synthetic.
Organic data describes information generated by actual humans, whether that’s a piece of writing, a numerical dataset, a song, an image, or a video. Synthetic data is created by generative AI models using organic data as a base material.
Synthetic Data and AI ‘Model Collapse’
Just as a photocopy of a photocopy can drift away from the original, when generative AI is trained on its own synthetic data, its output can also drift away from reality, growing further apart from the organic data that it was intended to imitate.
Transparency and Synthetic Data
The use of synthetic data isn’t inherently good or bad. In medical research, for example, it’s a critically important tool that allows scientists to make new discoveries while protecting the privacy of individual patients.
At the Transparency Coalition, we are not calling for limitations on the creation or use of synthetic data. What’s needed is disclosure: Developers should be transparent in their use of synthetic data when using it to train an AI model.
Training Data: What the Machine Learns
Training data is the foundation of artificial intelligence. It’s what AI systems like ChatGPT use to provide answers to the prompts we provide. It’s what generative image systems like Midjourney and DALL-E use to conjure AI-created art.
Why and How to Disclose the Use of AI
With the emergence of generative AI, it now takes just a few button-clicks for anyone to create or manipulate data and convince others that fake content is real.
Why Training Data Is Not a Trade Secret
Training data is the foundation of artificial intelligence. It’s what AI systems like ChatGPT use to provide answers to the prompts we provide. It’s what generative image systems like Midjourney and DALL-E use to conjure AI-created art.
Emerging Standards in Disclosure
Today most media/tech companies are coalescing around the standard created by the Coalition for Content Provenance and Authenticity (C2PA) .
How to Format and Require Data Disclosures
Developers of AI systems should be required to provide documentation for all training data used in the development of an AI model.
Legislating the Disclosure of AI Use
Legislative policies requiring the disclosure of the use of AI are developing side-by-side along the emerging standards in AI provenance. They’re not perfectly in sync—and that’s okay.
Data Privacy in the Age of AI
With the emergence of artificial intelligence systems like ChatGPT and CoPilot, data privacy has emerged as one of the most urgent consumer protection issues of the 2020s.
Why ‘Opt-in’ Consent Is the Best Option
Most state data privacy laws in the U.S. operate on an opt-out basis, meaning that users are assumed to have consented to the use of cookies unless they actively decline, ie, opt out.
Output Safeguards: Disclose the Use of AI
At the Transparency Coalition we believe the most important AI output provision is also the most basic: Disclose the use of AI.
California’s AI Transparency Act, adopted in Sept. 2024, provides a model for this kind of disclosure. The Act requires AI developers to embed detection tools in the media their AI creates, and to post an AI decoder tool on their website.
Defining Artificial Intelligence
AI computer systems are trained to think, learn, and make decisions independently. While common algorithms follow step-by-step instructions to solve specific tasks, AI systems can analyze data, recognize patterns, and improve their performance over time without explicit programming for every scenario.
How AI Systems Are Created
At its heart, an AI system is a highly sophisticated computer program. That program, known as a model, requires enormous amounts of computing power and massive datasets. By ingesting the datasets, the model “learns” about the structure of language, for instance, or patterns derived from millions of images.